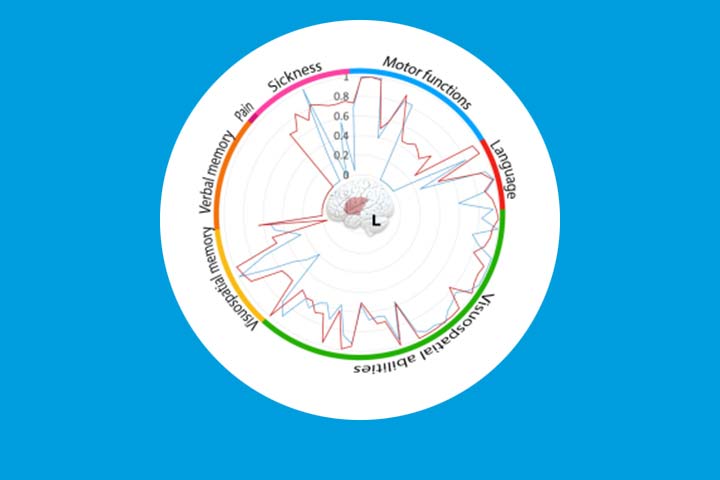
AI predicts recovery one year after stroke
Source: https://www.ru.nl/donders
‘Where will I stand in a year? Will I fully recover?’ These are the first questions asked by stroke patients. Based on data from nearly 2000 stroke survivors, this study published in Brain proposes a new artificial intelligence algorithm and web application that predicts the expected neuropsychological impact on patients one year after symptom onset.
Less than 30% returns to the workforce
Stroke affects one in six people at some point in their lives. Although not widely publicised, stroke is now the second leading cause of death worldwide and the most common cause of neurological disability in adults. Despite significant advances in therapies for stroke patients in recent decades, many will continue to have persistent motor and cognitive deficits affecting their quality of life, ability to communicate and socialise and return to work. It is estimated that less than 30% of patients return to the workforce, and even fewer returning to their pre-stroke occupation. Patient management could be optimised if more tailored and personalised tools were available to predict long-term outcomes. The standard clinical measures such as age, education, and initial impairment level used today are insufficient to make reliable predictions. Reliable predictions would reduce the burden and stress associated with a stroke while allowing patients to make the proper arrangements with their families, health insurance providers, and employers. In addition, it is essential for physicians and clinicians because it would allow a stratification of patients to optimise which treatment is given to whom and at what dose.
Predictive framework
Here we present a new predictive framework, the Disconnectome Symptoms Discoverer (http://disconnectomestudio.bcblab.com), to predict neuropsychological scores one year after stroke based on brain imaging and neuropsychological data from 2000 stroke patients. This scientific breakthrough was only possible because of the consideration of the brain’s organisation in white matter circuits, which had been neglected in earlier prediction analysis efforts (Thiebaut de Schotten & Forkel Science 2022). By linking this framework to neuropsychological findings, the authors obtained biological information available in the first comprehensive atlas of the relationships between brain circuit lesions and deficits on 86 neuropsychological scores – a neuropsychological atlas of white matter. The new predictive framework achieved better predictive performance than six other models, including functional disconnection, lesion topology and volume modelling. The out-of-sample prediction derived from this atlas had an average absolute error of less than 20% and allowed for personalised neuropsychological predictions. These predictions were also confirmed on an out-of-sample cohort for semantic fluency. In addition, model training and validation were also replicated in two external cohorts.
This framework is available as an interactive web application (http://disconnectomestudio.bcblab.com), to supply the basis for a new practical approach to modelling cognition in stroke. The atlas and web application will help reduce the burden associated with stroke by better managing the cognitive deficits of patients, the expectations of their families, and society at large while helping to tailor future personalised treatment programs and discover new therapeutic targets. The range of neuropsychological assessments and the predictive power of this new model will be further enriched in the future by involving patients and caregivers and encouraging participation through citizen science.
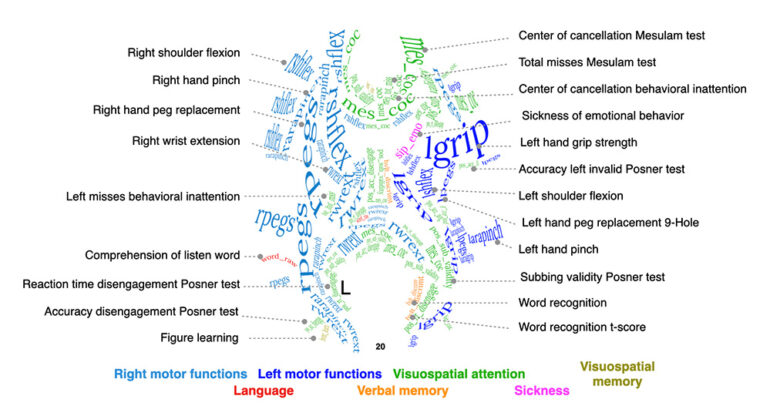
Référence de l’article
Latent disconnectome prediction of long-term cognitive-behavioural symptoms in stroke
Lia Talozzi, Stephanie J Forkel, Valentina Pacella, Victor Nozais, Etienne Allart, Céline Piscicelli, Dominic Pérennou, Daniel Tranel, Aaron Boes, Maurizio Corbetta, Parashkev Nachev, Michel Thiebaut de Schotten.
Brain, 16 March 2023.
DOI : https://doi.org/10.1093/brain/awad013
Contact
Michel Thiebaut de Schotten
Directeur de recherche CNRS
+33 (0)7 86 50 81 60
Last update 27/03/23