Validation of a Denoising Method Using Deep Learning-Based Reconstruction to Quantify Multiple Sclerosis Lesion Load on Fast FLAIR Imaging.
AJNR Am J Neuroradiol. 2022-07-28; 43(8): 1099-1106
DOI: 10.3174/ajnr.a7589
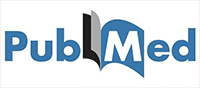
Read on PubMed
Yamamoto T(1), Lacheret C(2), Fukutomi H(1), Kamraoui RA(3), Denat L(1), Zhang B(4), Prevost V(5), Zhang L(6), Ruet A(7), Triaire B(5), Dousset V(1)(2)(8), Coupé P(3), Tourdias T(9)(2)(8).
Author information:
(1)From the Institut de Bio-imagerie (T.Y., H.F., L.D., V.D., T.T.), University
Bordeaux, Bordeaux, France.
(2)Neuroimagerie Diagnostique et Thérapeutique (C.L., V.D., T.T.).
(3)Laboratoire Bordelais de Recherche en Informatique (R.A.K., P.C.), University
Bordeaux, Le Centre National de la Recherche Scientifique, Bordeaux Institut
National Polytechnique, Talence, France.
(4)Canon Medical Systems Europe (B.Z.), Zoetermeer, the Netherlands.
(5)Canon Medical Systems (V.P., B.T.), Tochigi, Japan.
(6)Canon Medical Systems China (L.Z.), Beijing, China.
(7)Service de Neurologie (A.R.), Centre Hospitalier Universitaire de Bordeaux,
Bordeaux, France.
(8)NeurocentreMagendie (V.D., T.T.), University of Bordeaux, L’Institut National
de la Santé et de la Recherche Médicale, Bordeaux, France.
(9)From the Institut de Bio-imagerie (T.Y., H.F., L.D., V.D., T.T.), University
Bordeaux, Bordeaux, France .
BACKGROUND AND PURPOSE: Accurate quantification of WM lesion load is essential
for the care of patients with multiple sclerosis. We tested whether the
combination of accelerated 3D-FLAIR and denoising using deep learning-based
reconstruction could provide a relevant strategy while shortening the imaging
examination.
MATERIALS AND METHODS: Twenty-eight patients with multiple sclerosis were
prospectively examined using 4 implementations of 3D-FLAIR with decreasing scan
times (4 minutes 54 seconds, 2 minutes 35 seconds, 1 minute 40 seconds, and 1
minute 15 seconds). Each FLAIR sequence was reconstructed without and with
denoising using deep learning-based reconstruction, resulting in 8 FLAIR
sequences per patient. Image quality was assessed with the Likert scale,
apparent SNR, and contrast-to-noise ratio. Manual and automatic lesion
segmentations, performed randomly and blindly, were quantitatively evaluated
against ground truth using the absolute volume difference, true-positive rate,
positive predictive value, Dice similarity coefficient, Hausdorff distance, and
F1 score based on the lesion count. The Wilcoxon signed-rank test and 2-way
ANOVA were performed.
RESULTS: Both image-quality evaluation and the various metrics showed
deterioration when the FLAIR scan time was accelerated. However, denoising using
deep learning-based reconstruction significantly improved subjective image
quality and quantitative performance metrics, particularly for manual
segmentation. Overall, denoising using deep learning-based reconstruction helped
to recover contours closer to those from the criterion standard and to capture
individual lesions otherwise overlooked. The Dice similarity coefficient was
equivalent between the 2-minutes-35-seconds-long FLAIR with denoising using deep
learning-based reconstruction and the 4-minutes-54-seconds-long reference FLAIR
sequence.
CONCLUSIONS: Denoising using deep learning-based reconstruction helps to
recognize multiple sclerosis lesions buried in the noise of accelerated FLAIR
acquisitions, a possibly useful strategy to efficiently shorten the scan time in
clinical practice.
© 2022 by American Journal of Neuroradiology.
DOI: 10.3174/ajnr.A7589
PMCID: PMC9575422
PMID: 35902124 [Indexed for MEDLINE]