Model and experiments to optimize co-adaptation in a simplified myoelectric control system.
J. Neural Eng.. 2018-01-30; 15(2): 026006
DOI: 10.1088/1741-2552/aa87cf
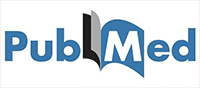
Read on PubMed
1. J Neural Eng. 2018 Apr;15(2):026006. doi: 10.1088/1741-2552/aa87cf.
Model and experiments to optimize co-adaptation in a simplified myoelectric
control system.
Couraud M(1), Cattaert D, Paclet F, Oudeyer PY, de Rugy A.
Author information:
(1)Institut de Neurosciences Cognitives et Intégratives d’Aquitaine, CNRS UMR
5287, Université de Bordeaux, France.
OBJECTIVE: To compensate for a limb lost in an amputation, myoelectric prostheses
use surface electromyography (EMG) from the remaining muscles to control the
prosthesis. Despite considerable progress, myoelectric controls remain markedly
different from the way we normally control movements, and require intense user
adaptation. To overcome this, our goal is to explore concurrent machine
co-adaptation techniques that are developed in the field of brain-machine
interface, and that are beginning to be used in myoelectric controls.
APPROACH: We combined a simplified myoelectric control with a perturbation for
which human adaptation is well characterized and modeled, in order to explore
co-adaptation settings in a principled manner.
RESULTS: First, we reproduced results obtained in a classical visuomotor rotation
paradigm in our simplified myoelectric context, where we rotate the muscle
pulling vectors used to reconstruct wrist force from EMG. Then, a model of human
adaptation in response to directional error was used to simulate various
co-adaptation settings, where perturbations and machine co-adaptation are both
applied on muscle pulling vectors. These simulations established that a
relatively low gain of machine co-adaptation that minimizes final errors
generates slow and incomplete adaptation, while higher gains increase adaptation
rate but also errors by amplifying noise. After experimental verification on real
subjects, we tested a variable gain that cumulates the advantages of both, and
implemented it with directionally tuned neurons similar to those used to model
human adaptation. This enables machine co-adaptation to locally improve
myoelectric control, and to absorb more challenging perturbations.
SIGNIFICANCE: The simplified context used here enabled to explore co-adaptation
settings in both simulations and experiments, and to raise important
considerations such as the need for a variable gain encoded locally. The benefits
and limits of extending this approach to more complex and functional myoelectric
contexts are discussed.
DOI: 10.1088/1741-2552/aa87cf
PMID: 28832013