Design of deep echo state networks
Neural Networks. 2018-12-01; 108: 33-47
DOI: 10.1016/j.neunet.2018.08.002
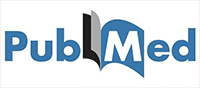
Read on PubMed
In this paper, we provide a novel approach to the architectural design of deep Recurrent Neural Networks using signal frequency analysis. In particular, focusing on the Reservoir Computing framework and inspired by the principles related to the inherent effect of layering, we address a fundamental open issue in deep learning, namely the question of how to establish the number of layers in recurrent architectures in the form of deep echo state networks (DeepESNs). The proposed method is first analyzed and refined on a controlled scenario and then it is experimentally assessed on challenging real-world tasks. The achieved results also show the ability of properly designed DeepESNs to outperform RC approaches on a speech recognition task, and to compete with the state-of-the-art in time-series prediction on polyphonic music tasks.