Shoulder kinematics plus contextual target information enable control of multiple distal joints of a simulated prosthetic arm and hand
J NeuroEngineering Rehabil. 2021-01-06; 18(1):
DOI: 10.1186/s12984-020-00793-0
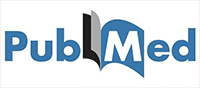
Lire sur PubMed
Background
Prosthetic restoration of reach and grasp function after a trans-humeral amputation requires control of multiple distal degrees of freedom in elbow, wrist and fingers. However, such a high level of amputation reduces the amount of available myoelectric and kinematic information from the residual limb.
Methods
To overcome these limits, we added contextual information about the target’s location and orientation such as can now be extracted from gaze tracking by computer vision tools. For the task of picking and placing a bottle in various positions and orientations in a 3D virtual scene, we trained artificial neural networks to predict postures of an intact subject’s elbow, forearm and wrist (4 degrees of freedom) either solely from shoulder kinematics or with additional knowledge of the movement goal. Subjects then performed the same tasks in the virtual scene with distal joints predicted from the context-aware network.
Results
Average movement times of 1.22s were only slightly longer than the naturally controlled movements (0.82 s). When using a kinematic-only network, movement times were much longer (2.31s) and compensatory movements from trunk and shoulder were much larger. Integrating contextual information also gave rise to motor synergies closer to natural joint coordination.
Conclusions
Although notable challenges remain before applying the proposed control scheme to a real-world prosthesis, our study shows that adding contextual information to command signals greatly improves prediction of distal joint angles for prosthetic control.