Application of Machine Learning to Arterial Spin Labeling in Mild Cognitive Impairment and Alzheimer Disease
Radiology. 2016-12-01; 281(3): 865-875
DOI: 10.1148/radiol.2016152703
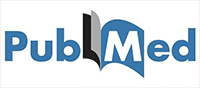
Lire sur PubMed
1. Radiology. 2016 Dec;281(3):865-875. Epub 2016 Jul 6.
Application of Machine Learning to Arterial Spin Labeling in Mild Cognitive
Impairment and Alzheimer Disease.
Collij LE(1), Heeman F(1), Kuijer JP(1), Ossenkoppele R(1), Benedictus MR(1),
Möller C(1), Verfaillie SC(1), Sanz-Arigita EJ(1), van Berckel BN(1), van der
Flier WM(1), Scheltens P(1), Barkhof F(1), Wink AM(1).
Author information:
(1)From the Department of Radiology and Nuclear Medicine (L.E.C., F.H., E.J.S.A.,
B.N.M.v.B., F.B., A.M.W.), Department of Physics and Medical Technology
(J.P.A.K.), and Alzheimer Centre and Department of Neurology (R.O., M.R.B., C.M.,
S.C.J.V., W.M.v.d.F., P.S.), VU University Medical Centre, Boelelaan 1118, 1081
HZ Amsterdam, the Netherlands.
Purpose To investigate whether multivariate pattern recognition analysis of
arterial spin labeling (ASL) perfusion maps can be used for classification and
single-subject prediction of patients with Alzheimer disease (AD) and mild
cognitive impairment (MCI) and subjects with subjective cognitive decline (SCD)
after using the W score method to remove confounding effects of sex and age.
Materials and Methods Pseudocontinuous 3.0-T ASL images were acquired in 100
patients with probable AD; 60 patients with MCI, of whom 12 remained stable, 12
were converted to a diagnosis of AD, and 36 had no follow-up; 100 subjects with
SCD; and 26 healthy control subjects. The AD, MCI, and SCD groups were divided
into a sex- and age-matched training set (n = 130) and an independent prediction
set (n = 130). Standardized perfusion scores adjusted for age and sex (W scores)
were computed per voxel for each participant. Training of a support vector
machine classifier was performed with diagnostic status and perfusion maps.
Discrimination maps were extracted and used for single-subject classification in
the prediction set. Prediction performance was assessed with receiver operating
characteristic (ROC) analysis to generate an area under the ROC curve (AUC) and
sensitivity and specificity distribution. Results Single-subject diagnosis in the
prediction set by using the discrimination maps yielded excellent performance for
AD versus SCD (AUC, 0.96; P < .01), good performance for AD versus MCI (AUC,
0.89; P < .01), and poor performance for MCI versus SCD (AUC, 0.63; P = .06).
Application of the AD versus SCD discrimination map for prediction of MCI
subgroups resulted in good performance for patients with MCI diagnosis converted
to AD versus subjects with SCD (AUC, 0.84; P < .01) and fair performance for
patients with MCI diagnosis converted to AD versus those with stable MCI (AUC,
0.71; P > .05). Conclusion With automated methods, age- and sex-adjusted ASL
perfusion maps can be used to classify and predict diagnosis of AD, conversion of
MCI to AD, stable MCI, and SCD with good to excellent accuracy and AUC values. ©
RSNA, 2016.
DOI: 10.1148/radiol.2016152703
PMID: 27383395 [Indexed for MEDLINE]