A review of user training methods in brain computer interfaces based on mental tasks
J. Neural Eng.. 2021-02-01; 18(1): 011002
DOI: 10.1088/1741-2552/abca17
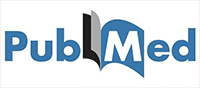
Lire sur PubMed
Roc A(1), Pillette L(1), Mladenovic J(1), Benaroch C(2), N’Kaoua B(3), Jeunet C(4), Lotte F(1).
Author information:
(1)Inria Bordeaux, Talence, FRANCE.
(2)Inria Centre de recherche Bordeaux Sud-Ouest, Talence, 33405, FRANCE.
(3)Handicap, Activity, Cognition, Health, Inserm / University of Bordeaux, Talence, FRANCE.
(4)CLLE, Toulouse, Occitanie, FRANCE.
Mental-Tasks based Brain-Computer Interfaces (MT-BCIs) allow their users to interact with an external device solely by using brain signals produced through
mental tasks. While MT-BCIs are promising for many applications, they are still barely used outside laboratories due to their lack of reliability. MT-BCIs
require their users to develop the ability to self-regulate specific brain signals. However, the human learning process to control a BCI is still relatively
poorly understood and how to optimally train this ability is currently under investigation. Despite their promises and achievements, traditional training
programs have been shown to be sub-optimal and could be further improved. In order to optimize user training and improve BCI performance, human factors should be taken into account. An interdisciplinary approach should be adopted to provide learners with appropriate and/or adaptive training. In this article, we provide an overview of existing methods for MT-BCI user training – notably in terms of environment, instructions, feedback and exercises. We present a categorization and taxonomy of these training approaches, provide guidelines on how to choose the best methods and identify open challenges and perspectives to further improve MT-BCI user training.