A novel group ICA approach based on multi-scale individual component clustering. application to a large sample of fMRI data
Neuroinform. 2012-03-18; 10(3): 269-285
DOI: 10.1007/s12021-012-9145-2
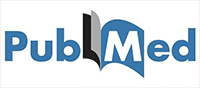
Lire sur PubMed
1. Neuroinformatics. 2012 Jul;10(3):269-85. doi: 10.1007/s12021-012-9145-2.
A novel group ICA approach based on multi-scale individual component clustering.
Application to a large sample of fMRI data.
Naveau M(1), Doucet G, Delcroix N, Petit L, Zago L, Crivello F, Jobard G, Mellet
E, Tzourio-Mazoyer N, Mazoyer B, Joliot M.
Author information:
(1)Univ. de Bordeaux, GIN, UMR 5296, Bordeaux, France.
Functional connectivity-based analysis of functional magnetic resonance imaging
data (fMRI) is an emerging technique for human brain mapping. One powerful method
for the investigation of functional connectivity is independent component
analysis (ICA) of concatenated data. However, this research field is evolving
toward processing increasingly larger database taking into account
inter-individual variability. Concatenated data analysis only handles these
features using some additional procedures such as bootstrap or including a model
of between-subject variability during the preprocessing step of the ICA. In order
to alleviate these limitations, we propose a method based on group analysis of
individual ICA components, using a multi-scale clustering (MICCA). MICCA start
with two steps repeated several times: 1) single subject data ICA followed by 2)
clustering of all subject independent components according to a spatial
similarity criterion. A final third step consists in selecting reproducible
clusters across the repetitions of the two previous steps. The core of the
innovation lies in the multi-scale and unsupervised clustering algorithm built as
a chain of three processes: robust proto-cluster creation, aggregation of the
proto-clusters, and cluster consolidation. We applied MICCA to the analysis of
310 fMRI resting state dataset. MICCA identified 28 resting state brain networks.
Overall, the cluster neuroanatomical substrate included 98% of the cerebrum gray
matter. MICCA results proved to be reproducible in a random splitting of the data
sample and more robust than the classical concatenation method.
DOI: 10.1007/s12021-012-9145-2
PMID: 22426994 [Indexed for MEDLINE]